Mingda Li: Phonon Machine Learning
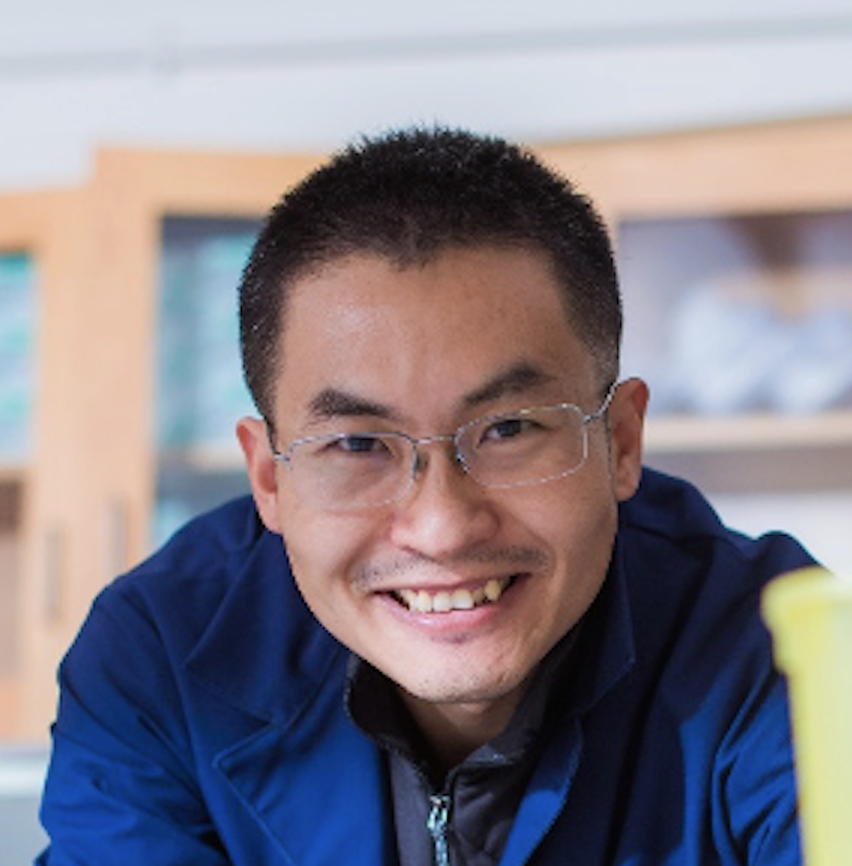
Abstract:
Phonons are the collective lattice vibrations and play a major role in materials thermal properties. However, phonon properties are often challenging to obtain with either high computational cost of complex experimental setup. In this seminar, after a pedagogical introduction on graph neural networks, we introduce three of our recent efforts aiming to acquire phonon properties. First, we introduce an end-to-end predictor that takes in crystal structures as input and outputs phonon density-of-states curve [1]. With small volume of training data, symmetry argumentation in a graph neural network plays an essential role. Second, we introduce an integrated experimental-computational framework that can extract frequency-resolve phonon transmittance and relaxation time. Ultrafast diffraction offers high-dimensional data in time-momentum space where phonon thermal transport is reflected on the atomic vibrations and thereby diffraction intensities, and data-free neural ODE is used to solve an inverse thermal transport problem and extract those frequency-resolved phonon transport information [2]. Finally, we introduce our latest work on predicting phonon dispersion relation, by designing the virtual node graph networks [3]. The method enables the introduction of reciprocal space in real-space graph neural networks, and allows prediction of materials properties with variable length. With addition anharmonic calculations, the lattice thermal conductivity and thermal expansion can also be predicted.
[1] Z Chen, N Andrejevic, T Smidt, M Li, “Direct prediction of phonon density of states with Euclidean neural networks”, Adv. Sci. 8, 2004214 (2021).
[2] Z Chen, X Shen, M Li, Panoramic mapping of phonon transport from ultrafast electron diffraction and machine learning", Advanced Materials 35, 2206997 (2023).
[3] R Okabe, A Chotrattanapituk, B Liao, YQ Cheng, M Li, "Virtual Node Graph Neural Network for Full Phonon Prediction", arXiv:2301.02197 (2023).
Bio:
Mingda is the Class of 1947 Career Development Professor, and an Associate Professor of Nuclear Science and Engineering at MIT. Mingda’s research is composed of three parts, including 1) thermal transport, such as learning and measuring phonon properties, 2) topological quantum materials, synthesizing new topological materials toward energy harvesting applications, and 3) fundamental principles of measurement, to answer what makes a quantity measurable and how to uncover hidden materials properties by designing new measurement paradigms.